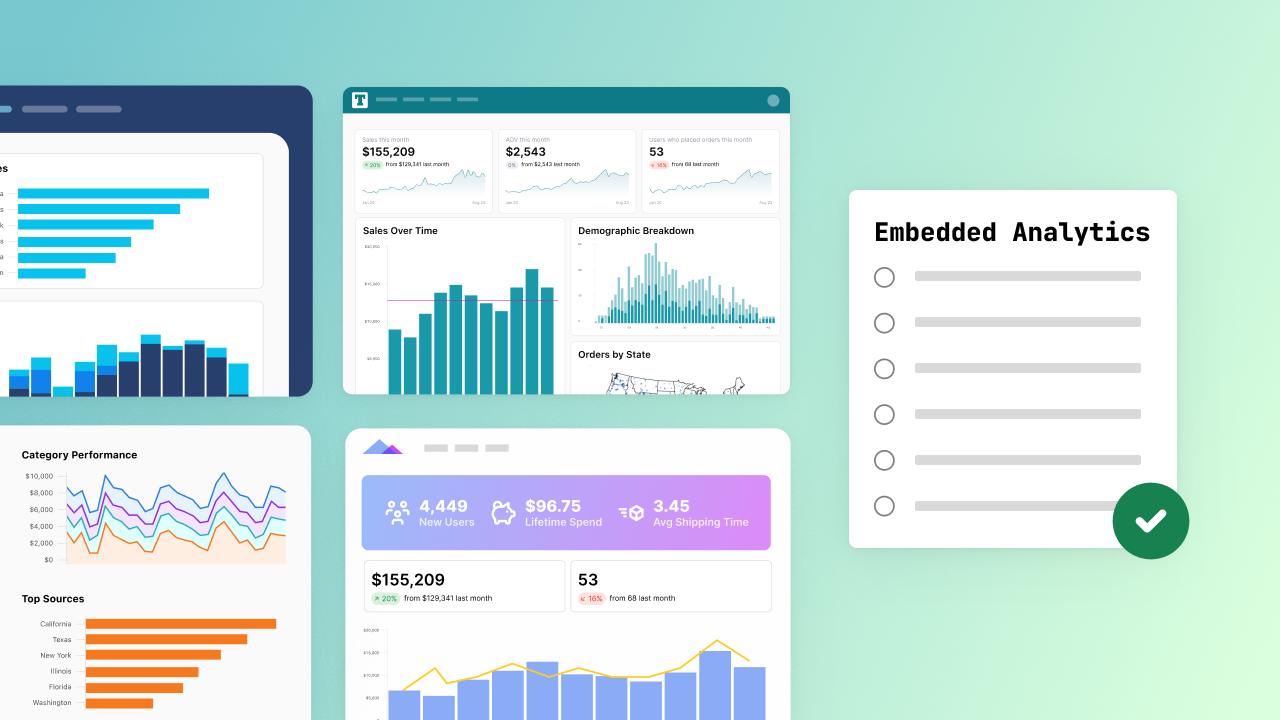
The novelty of data products has worn off. Conversations have shifted from whether or not to build data products to how to build them more efficiently and create more customer value.
It makes sense; the stakes are high when building customer-facing data products. This is why more teams have shifted to solving this problem by partnering with an embedded analytics provider. Rather than spending months (or years) trying to ship V0 of a custom, in-house product, it's often much more efficient to leverage the infrastructure, expertise, and features of an existing solution.
But how do you choose?
A lot has changed since the legacy players first launched, and you shouldn’t be forced to compromise. It's important to find an embedded analytics partner who will meet your needs today and has a roadmap that meets your long-term vision. To help you evaluate the right fit for your business, here are six key criteria to consider:
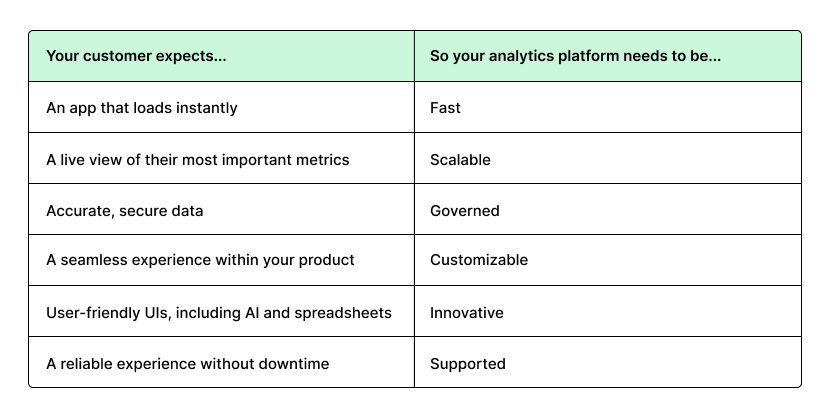
Consideration #1: Speed #

Speed is essential for a great user experience. When evaluating analytics platforms, focus on architecture that ensures fast performance and fresh data. A cloud-based tool with automatic caching can help users get real-time answers and prevent unnecessary trips to your database. For example, Omni has a built-in intelligent cache – it leverages results from recent queries in the cache instead of sending new requests down to your database, delivering results fast while helping keep your compute costs low.
Questions to help you evaluate for speed:
Does it enable your product(s) to meet the speed and reliability standards of your team and customers?
Does it allow you to build products that actually improve the user experience, or does slow performance detract or distract users?
Is the tool architected to limit trips to the data warehouse?
Consideration #2: Scalability #
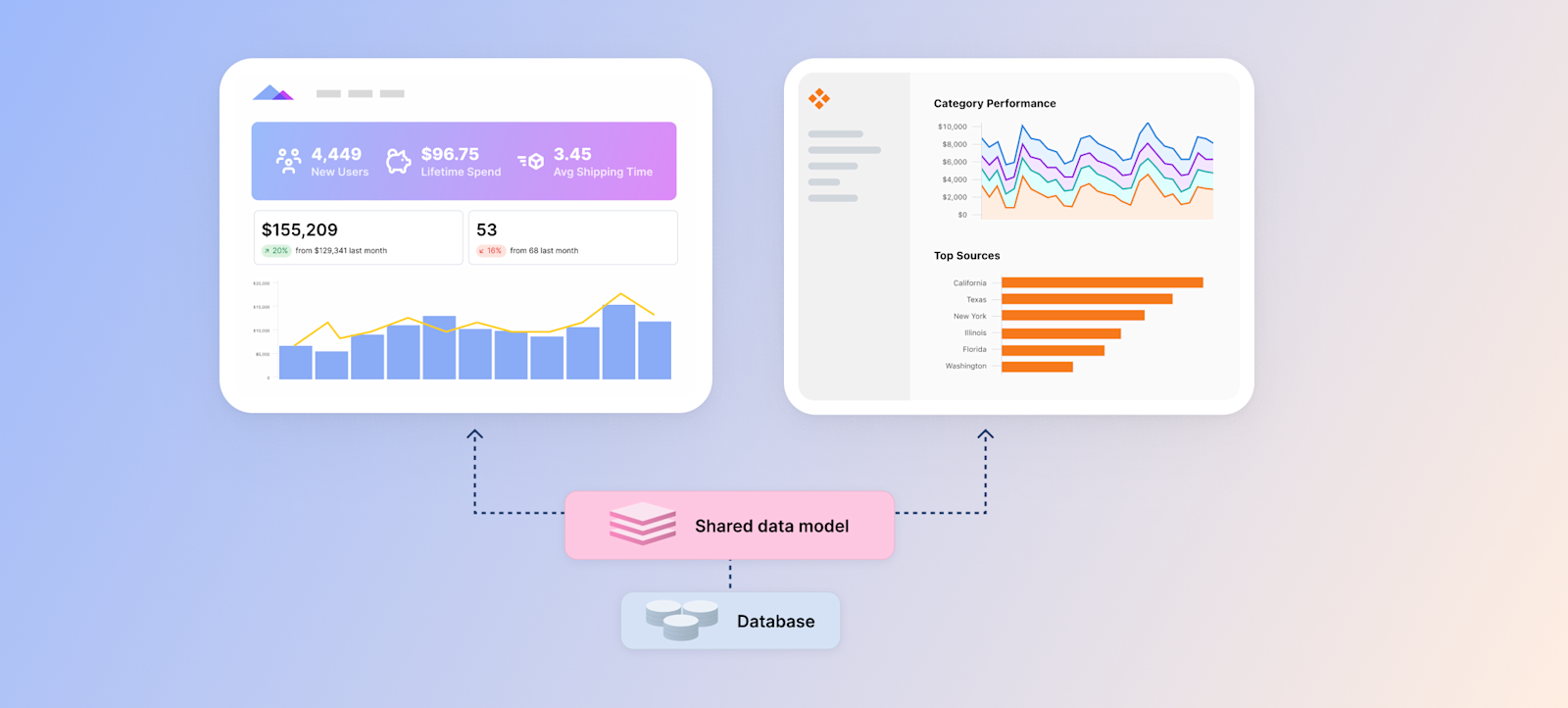
Data products have become more widely accessed than most of the BI tools that are powering them. While most legacy BI tools have added features to enable embedded analytics, they weren’t built to manage the scale that today’s use cases demand.
Building a data product from scratch takes significant engineering time to develop, maintain, and de-bug – especially as your user base grows and your product becomes more complex. Many of our customers who migrated from homegrown solutions found their previous experience building data products from scratch took far more time than expected, often pulling resources away from core product development.
As your user base grows, your analytics platform needs to grow with it. A shared data model can be a game-changer, helping you scale efficiently while avoiding maintenance headaches. This is because a shared data model allows you to make updates in one central place and proliferate those changes to all customer-facing reports simultaneously. Instead of spending hours hunting down specific reports or dashboards, you can make changes in bulk to reduce the burden of maintenance.
A flexible development UI will also help you scale. Whether your data team, product team, customer success team, or a mixture of teams is building your data product, having deep integrations with other data tools and a UI with intuitive point & click options is critical for long-term scalability. To see how this looks in action, check out this on-demand session where our CTO, Chris, and Solutions Engineer, Jack, built a fully functional data product in under 30 minutes with a live audience.
Questions to help you evaluate for scalability:
How do I expect these products to grow and scale over time? How many users do we hope to support in one year, in five years, in ten years?
What is the change-management process like with this tool? When I publish a change, how will I know that change is reflected in all the right places and guard against breaks?
How quickly is my team able to build with this tool? And how does the tool help prevent duplicative work?
Consideration #3: Governance #
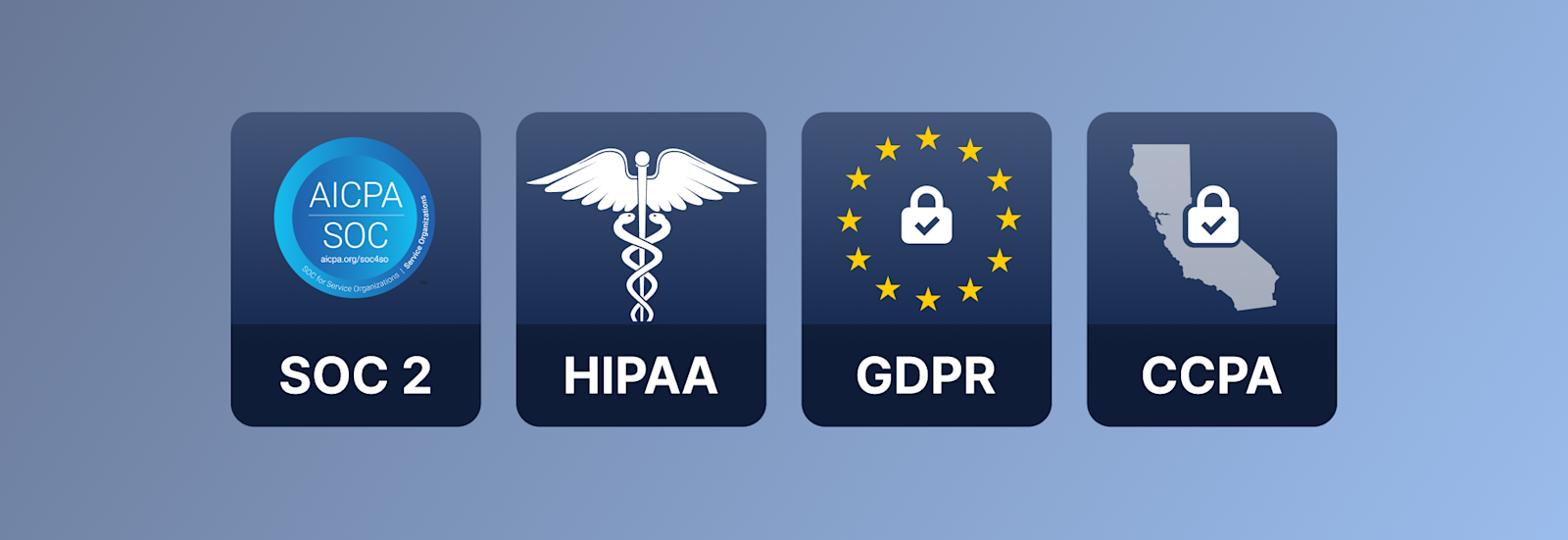
Governance – ensuring users see the correct data – is uniquely important for customer-facing analytics products. However, governance for data products can be even trickier than standard BI; the number of users may outnumber the number of people in your company, and you don’t know as much about their workflows as you do for internal use cases.
Start by ensuring your analytics provider makes it simple to manage data permissions and comply with standards like SOC 2, HIPAA, and GDPR. A shared data model also helps by centralizing user access, permissions, and change monitoring.
Questions to help you evaluate for governance:
What access and administrative controls are available? Are they automatically included in the contract, or do you have to pay more for access to these features?
What features and workflows are in place to help alignment for product and engineering teams?
How does the tool deal with change-management (e.g. Git integrations and code-based version control)?
Consideration #4: Customizability #
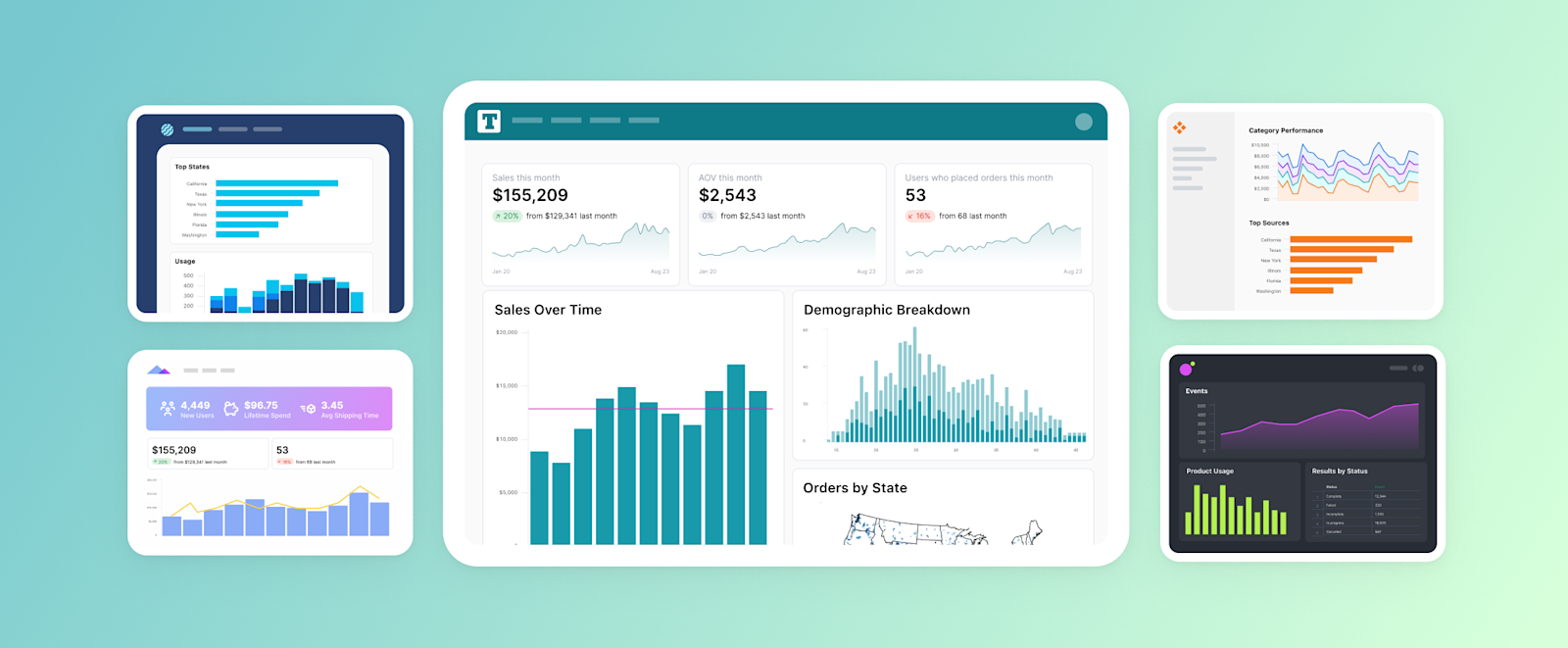
This is where your search gets more fun. Your product’s design sets the tone for your customer experience. The right analytics platform should seamlessly fit within that design instead of feeling like it was bolted on.
Customization is often one of the hardest things to control when deciding between an embedded analytics solution vs. an in-house solution. So many font and color choices went into making your app look the way it does, and the provider you choose should make it easy for you to build your brand, or your customer’s brand, into the data elements of your product. For example, PrimaryBid built a portal unique to each of its customers’ brands, while integrating seamlessly with the rest of its application.
Questions to help you evaluate for customizability:
What level of customization would facilitate the customer experience you want for your data product?
As you consider different tools, what level of customization is possible? Does it meet those requirements?
How much customization is available out of the box vs. via API? How long would it take your team to build customer-facing reports?
If you were to build the product internally, how many engineering hours do you estimate it would take?
Consideration #5: Innovation #
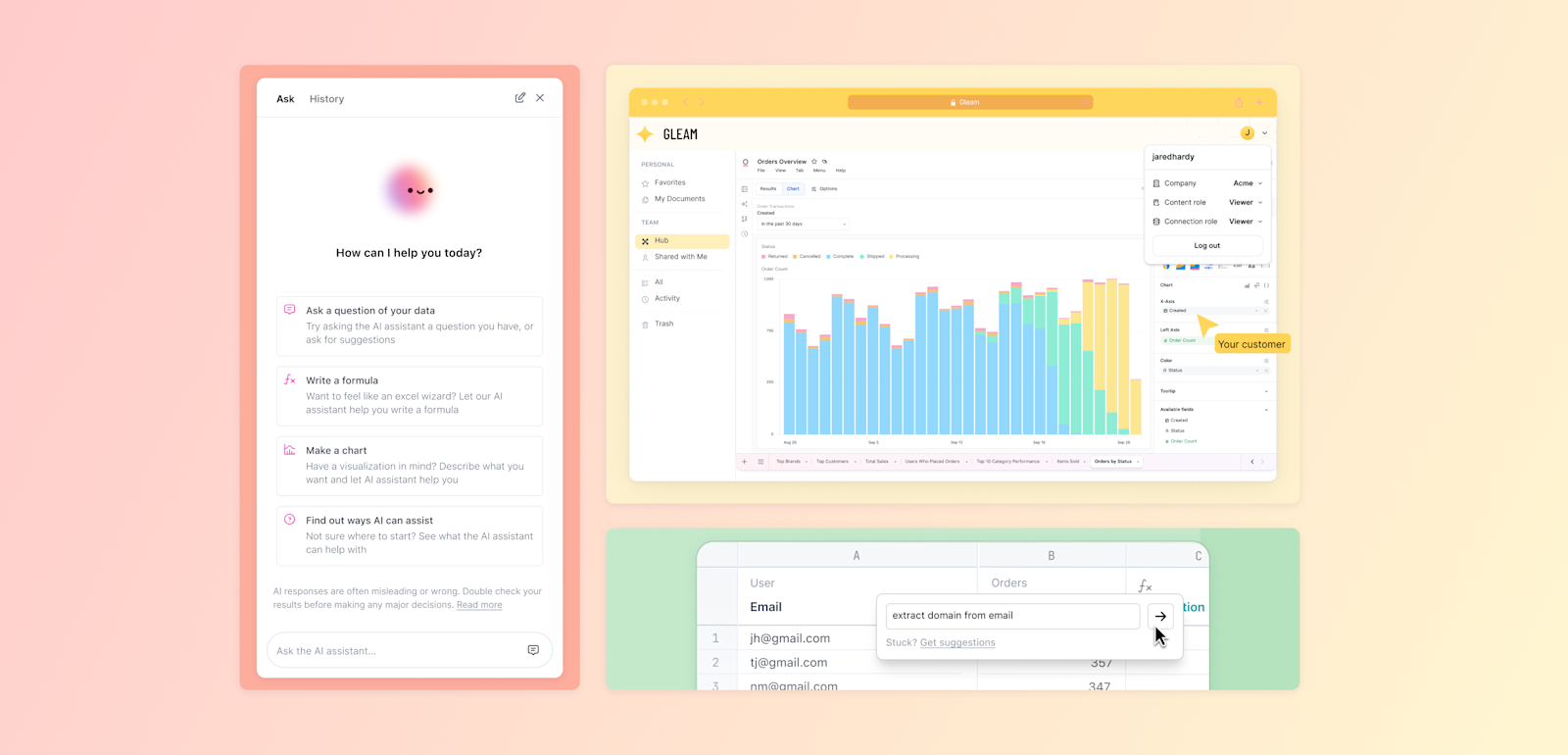
BI tools are often built for data experts, but your data product may not be. If you want your customers to engage with data and not just consume it, your data product should offer ways to interact with data that cater to a wide range of preferences and skills. And as those preferences and skills change, you should be able to rely on your analytics partner to innovate and ship new features quickly.
For example, Excel syntax can feel most familiar for finance and business users, while SQL can be faster and easier for engineers. And today, everyone expects data products to offer AI-supported querying or natural language queries.
Since your team is constantly innovating for your customers, the analytics provider behind your data product should be too. No part of your product should make your customers feel like they’re compromising.
Questions to help you evaluate for innovation:
Do your customers need to self-serve and create their own reports?
What are the self-service components of your product(s)? Will users feel comfortable interacting with data?
What types of users will be leveraging the products? How would they be most comfortable working or building?
Does the tool enable multiple development experiences or workflows?
How often does the tool ship new features? What does the roadmap look like for the future?
Consideration #6: Support #
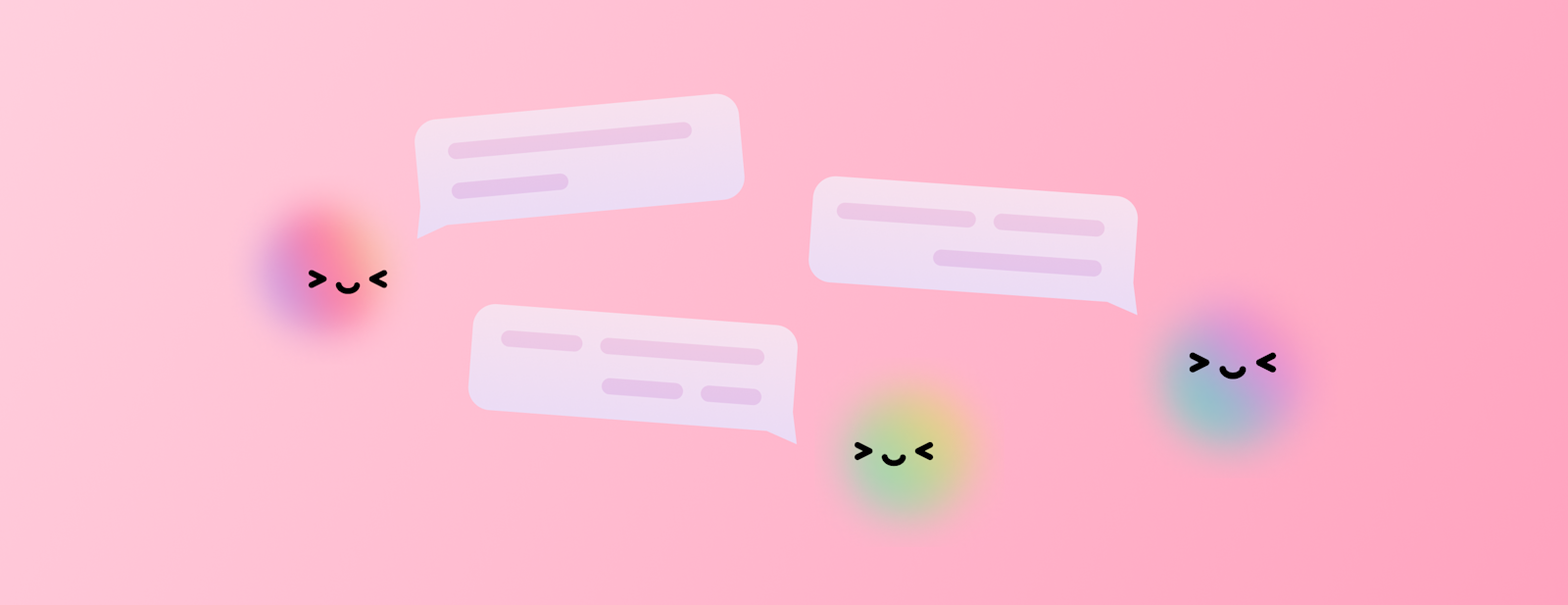
Customer support might not top your list of criteria, but it’s critical. With any BI tool, you’re choosing both a product and a team. The right team can be as vital as the tool itself, offering the expertise and readiness you need to succeed.
Since they’re helping you build your own product and provide a great experience to your customers, you need to ensure that they take that privilege seriously. At Omni, customer support is one of our top priorities. This means our entire company – from our CEO to our engineers – provides white-glove support and helps our customers meet their goals.
Questions to help you evaluate for support:
What will support look like during and after the POC? How can I get in touch with your team when I have questions or feedback? How responsive is the team when I reach out?
What SLAs does your team have regarding support tickets and general questions?
How big is your team? How many accounts is each rep managing?
Do I have access to your executives/leadership and product team for direct feedback?
Does the pricing model align and scale with our business model?
Choosing the right embedded analytics platform #
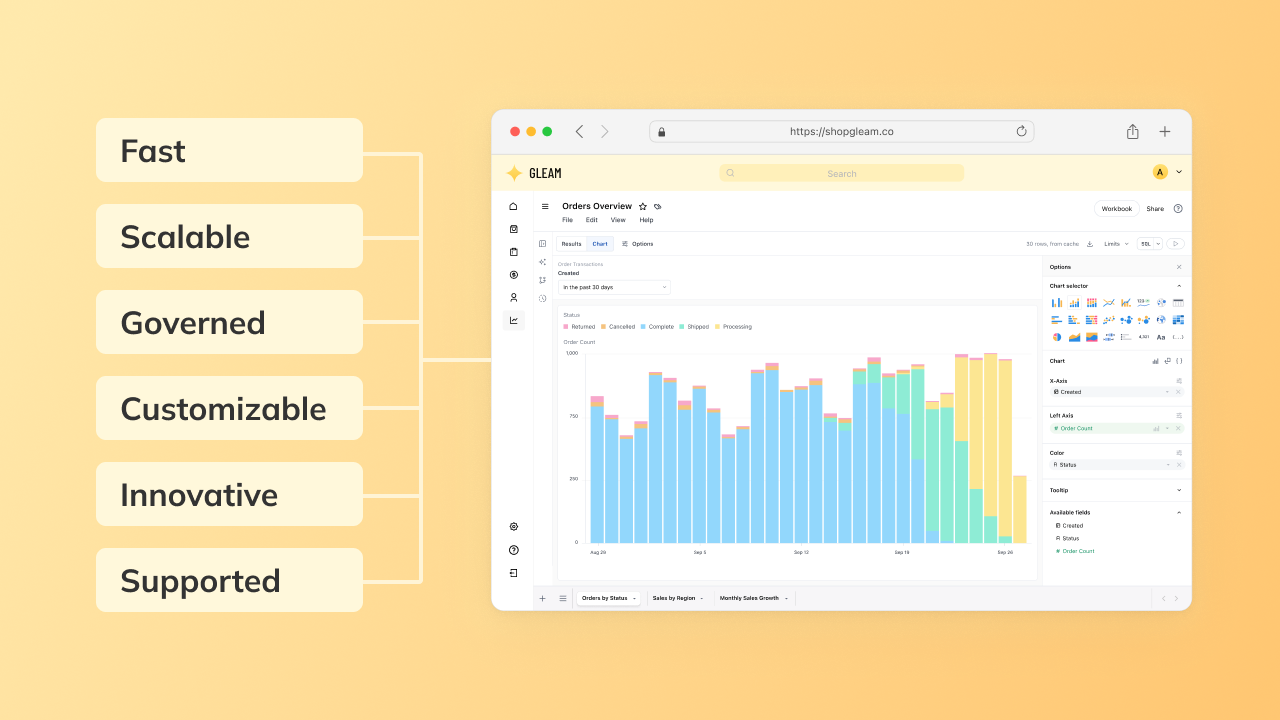
For an embedded analytics offering to be truly valuable, it should provide a great experience for your customers and a great development experience for your team. With careful consideration, the right technology platform will help you achieve the customer experience (and revenue goals) you want.
We built Omni to solve customer challenges for both embedded and internal analytics, and we’ve met with hundreds of teams assessing both areas. We’d love to show you more.